Expertises
Computer Science
- Models
- Explainable Artificial Intelligence
- Chain Rule
- Prediction Model
- Preoperative Data
- Machine Learning
Medicine and Dentistry
- Hip Fracture
- Surgical Mortality
Organisaties
Publicaties
2024
Feature Importance to Explain Multimodal Prediction Models: a Clinical Use Case (2024)In Explainable Artificial Intelligence: Second World Conference, xAI 2024. Valletta, Malta, July 17-19, 2024. Proceedings, Part IV (pp. 84-101) (Communications in Computer and Information Science; Vol. 2156). Springer Nature. van de Beld, J.-J., Pathak, S., Geerdink, J., Hegeman, J. H. & Seifert, C.https://doi.org/10.1007/978-3-031-63803-9_5Prototype-Based Interpretable Breast Cancer Prediction Models: Analysis and Challenges (2024)In Explainable Artificial Intelligence - 2nd World Conference, xAI 2024, Proceedings (pp. 21-42) (Communications in Computer and Information Science; Vol. 2153 CCIS). Springer. Pathak, S., Schlötterer, J., Veltman, J., Geerdink, J., van Keulen, M. & Seifert, C.https://doi.org/10.1007/978-3-031-63787-2_2Feature importance to explain multimodal prediction models. A clinical use case (2024)[Working paper › Preprint]. ArXiv.org. van de Beld, J.-J., Pathak, S., Geerdink, J., Hegeman, J. H. & Seifert, C.Prototype-based Interpretable Breast Cancer Prediction Models: Analysis and Challenges (2024)[Working paper › Preprint]. Pathak, S., Schlötterer, J., Veltman, J., Geerdink, J., Keulen, M. v. & Seifert, C.
2023
From Anecdotal Evidence to Quantitative Evaluation Methods: A Systematic Review on Evaluating Explainable AI (2023)ACM computing surveys, 55(13s). Article 295. Nauta, M., Trienes, J., Pathak, S., Nguyen, E., Peters, M., Schmitt, Y., Schlötterer, J., Van Keulen, M. & Seifert, C.https://doi.org/10.1145/3583558Weakly Supervised Learning for Breast Cancer Prediction on Mammograms in Realistic Settings (2023)[Working paper › Preprint]. ArXiv.org. Pathak, S., Schlötterer, J., Geerdink, J., Vijlbrief, O. D., Keulen, M. v. & Seifert, C.Benchmarking eXplainable AI: A Survey on Available Toolkits and Open Challenges (2023)In Proceedings of the 32nd International Joint Conference on Artificial Intelligence, IJCAI 2023 (pp. 6665-6673) (IJCAI International Joint Conference on Artificial Intelligence; Vol. 2023-August). International Joint Conferences on Artificial Intelligence. Le, P. Q., Nauta, M., Nguyen, V. B., Pathak, S., Schlötterer, J. & Seifert, C.
2022
Channel Contribution In Deep Learning Based Automatic Sleep Scoring – How Many Channels Do We Need? (2022)IEEE transactions on neural systems and rehabilitation engineering, 31, 494-505. Lu, C., Pathak, S., Englebienne, G. & Seifert, C.https://doi.org/10.1109/TNSRE.2022.3227040From Anecdotal Evidence to Quantitative Evaluation Methods: A Systematic Review on Evaluating Explainable AI (2022)[Working paper › Preprint]. ArXiv.org. Nauta, M., Trienes, J., Pathak, S., Nguyen, E., Peters, M., Schmitt, Y., Schlötterer, J., van Keulen, M. & Seifert, C.https://doi.org/10.48550/arXiv.2201.08164Multimodal Machine Learning for 30-Days Post-Operative Mortality Prediction of Elderly Hip Fracture Patients (2022)In Proceedings - 21st IEEE International Conference on Data Mining Workshops, ICDMW 2021 (pp. 508-516) (IEEE International Conference on Data Mining Workshops, ICDMW; Vol. 2021-December). IEEE. Yenidogan, B., Pathak, S., Geerdink, J., Hegeman, J. H. & Van Keulen, M.https://doi.org/10.1109/ICDMW53433.2021.00068
Onderzoeksprofielen
Adres
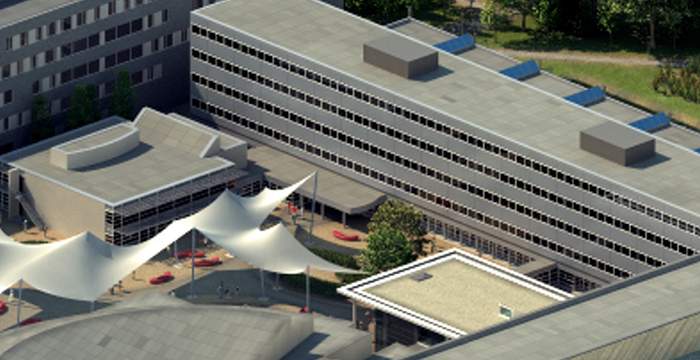
Universiteit Twente
Zilverling (gebouwnr. 11), kamer 4057
Hallenweg 19
7522 NH Enschede
Universiteit Twente
Zilverling 4057
Postbus 217
7500 AE Enschede